COMPARISONS of DM Tool Discovery
with other well known DM methods
Financial forecasting
The results of "Discovery" Tool comparisons with Neural
Networks (NN), Decision trees (Sipina), Rules extracted from NN,
First-order logic methods (FOIL) and other benchmark methods presented
on the table and figure
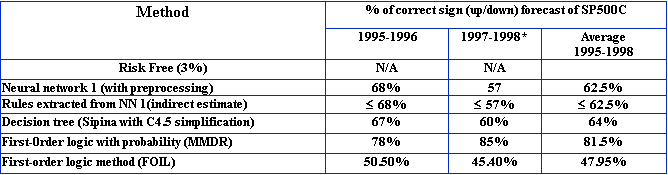
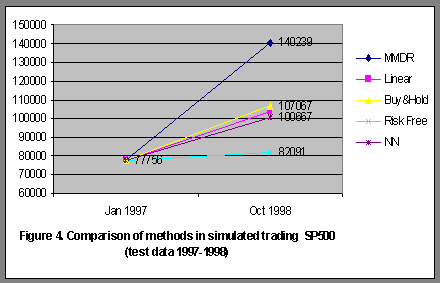
Breast cancer diagnostic system
The results of comparisons with Neural Networks, Decision
Tree (SIPINA), Linear Discriminant Analysis, "SIGAMD" software
Figure presents results
for another selection criterion: level of conditional probability. We studied
three levels: 0.7, 0.85 and 0.95. A higher level of conditional probability
decreases the number of rules and diagnosed patients, but increases accuracy of
diagnosis. Results for them are marked as MMDR1, MMDR2 and MMDR3. We extracted
44 statistically significant diagnostic rules for 0.05 level of F –criterion
with a conditional probability no less than 0.75 (MMDR1). There were 30 rules
with a conditional probability no less than 0.85 (MMDR2) and 18 rules with a
conditional probability no less than 0.95 (MMDR3). The total accuracy of
diagnosis is 82%. The false negative rate was 6.5% (9 malignant cases were
diagnosed as benign) and the false positive rate was 11.9% (16 benign cases
were diagnosed as malignant). The most reliable 30 rules delivered a total
accuracy of 90%, and the 18 most reliable rules performed with 96.6% accuracy
with only 3 false positive cases (3.4%). Neural Network (“Brainmaker”, California
Scientific Software) software had given 100% accuracy on training data, but for
the Round-Robin test, the total accuracy fell to 66%. The main reason for this
low accuracy is that Neural Networks (NN) do not evaluate the statistical
significance of the perfect performance (100%) on training data. Poor results
(76% on training data test) were also obtained with Linear Discriminant
Analysis (“SIGAMD” software, StatDialogue software, Moscow). The Decision Tree
approach (“SIPINA” software, Universite Lumiere, Lyon, France) performed with
accuracy of 76%-82% on training data. This is worse than what we obtained for
the MMDR method with the much more difficult Round-Robin test (fig. 8). The
very important false-negative rate was 3-8 cases (MMDR), 8-9 cases (Decision
Tree), 19 cases (Linear Discriminant Analysis) and 26 cases (NN).
In these experiments, rule-based methods (MMDR and decision trees) outperformed other methods.
Note also that only MMDR and decision trees produce diagnostic rules. These rules make
a computer-aided diagnostic decision process visible, transparent to
radiologists. With these methods radiologists can control and evaluate the
decision making process. Linear discriminant analysis gives an equation, which
separates benign and malignant classes. For example, 0.0670x1-0.9653x2+…
represents a case. How would one interpret a weighted number of
calcifications/cm 2 (0.0670x1) plus a weighted volume (cm 3), i.e., 0.9653x2?
There is no direct medical sense in this arithmetic.
|